NVIDIA grabbed the spotlight at AWS re:Invent by introducing NVIDIA NeMo Retriever, a new generative AI microservice promising to improve the enterprise AI landscape by enabling the integration of custom large language models (LLMs) with enterprise data. The goal is for NeMo Retriever to deliver unprecedented accurate responses for AI applications in the enterprise.
NVIDIA grabbed the spotlight at AWS re:Invent by introducing NVIDIA NeMo Retriever, a new generative AI microservice promising to improve the enterprise AI landscape by enabling the integration of custom large language models (LLMs) with enterprise data. The goal is for NeMo Retriever to deliver unprecedented accurate responses for AI applications in the enterprise.
NVIDIA NeMo Retriever is the latest addition to the NeMo family, known for its advanced frameworks and tools designed to build, customize, and deploy cutting-edge generative AI models. This enterprise-grade semantic-retrieval microservice is engineered to enhance generative AI applications with robust retrieval-augmented generation (RAG) capabilities.
What sets NeMo Retriever apart is its ability to provide more accurate responses through NVIDIA-optimized algorithms. This microservice allows developers to seamlessly connect their AI applications to diverse business data, irrespective of location- across clouds or in data centers. A part of the NVIDIA AI Enterprise software platform, it is conveniently available in the AWS Marketplace.
NVIDIA NeMo Retriever Already In Use
The technology’s impact is already felt with industry leaders like Cadence, Dropbox, SAP, and ServiceNow collaborating with NVIDIA. They are integrating this technology into their custom generative AI applications and services, pushing the boundaries of what’s possible in business intelligence.
“Generative AI introduces innovative approaches to address customer needs, such as tools to uncover potential flaws early in the design process,” Anirudh Devgan, president and CEO of Cadence
Cadence, a global leader in electronic systems design, is harnessing NeMo Retriever to develop RAG features for AI applications in industrial electronics design. Anirudh Devgan, Cadence’s CEO, highlighted the potential of generative AI in uncovering design flaws early, thereby accelerating high-quality product development.
Unlike open-source RAG toolkits, NeMo Retriever is coming to market with its production-ready stance, offering commercially viable models, API stability, security patches, and enterprise support. Its optimized embedding models are a major technological advancement, capturing intricate word relationships and enhancing LLMs’ processing and analysis capabilities.
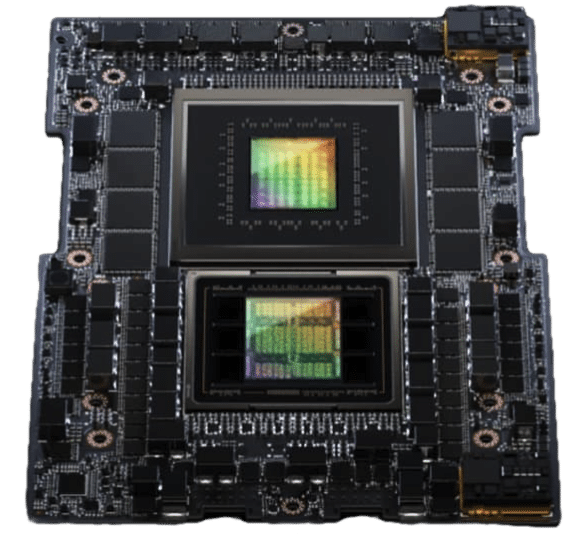
NVIDIA GH200 Superchip
Why NVIDIA NeMo Retriever Matters
NeMo Retriever’s ability to connect LLMs to multiple data sources and knowledge bases is remarkable. It enables users to interact with data through simple conversational prompts, obtaining accurate, up-to-date responses. This functionality extends across various data modalities, including text, PDFs, images, and videos, ensuring comprehensive and secure information access.
Most excitingly, NVIDIA NeMo Retriever promises more accurate results with less training, which speeds up the time to market and supports energy efficiency in the development workflow of generative AI applications.
This is where NeMo Retriever really shines. By integrating RAG with LLMs, NeMo Retriever overcomes the limitations of traditional models. RAG combines the power of information retrieval with LLMs, particularly for open-domain question-answering applications, thus significantly enhancing the LLMs’ access to vast, updateable knowledge bases.
A Look Into the RAG Pipeline
NeMo Retriever optimizes RAG processes, starting from encoding the knowledge base in an offline phase. In this phase, documents in various formats are chunked and embedded using a deep-learning model to produce dense vector representations. These are then stored in a vector database, crucial for semantic searches later. The embedding process is key, as it captures the relationships between words, enabling LLMs to process and analyze textual data with enhanced accuracy.
Under the hood, in production, and answering questions is where NeMo Retriever’s capabilities genuinely shine. It involves two crucial phases: retrieval from the vector database and response generation. When a user inputs a query, NeMo Retriever first embeds this query as a dense vector, using it to search the vector database. This database then retrieves the most relevant document chunks related to the query. In the final phase, these chunks are combined to form a context, which, along with the user’s query, is fed into the LLM. This process ensures that the responses generated are accurate and highly relevant to the user’s query.
Overcoming Enterprise Challenges with NVIDIA NeMo
Building a RAG pipeline for enterprise applications is a significant challenge. From the complexity of real-world queries to the demand of multi-turn conversations, enterprises require a solution that is both technologically advanced, compliant, and commercially viable. NeMo Retriever aims to fulfil this task by providing production-ready components optimized for low latency and high throughput, promising that enterprises can deploy these capabilities in their AI applications.
NVIDIA’s commitment to this technology extends to continuously improving its models and services, as with the NVIDIA Q&A Retrieval Embedding Model. As a transformer encoder, NVIDIA says it has been fine-tuned to provide the most accurate embeddings for text-based question-answering, leveraging both private and public datasets.
Empowering Enterprises with State-of-the-Art AI
The practical applications of NeMo Retriever are vast and varied. From IT and HR help assistants to R&D research assistants, NeMo Retriever’s ability to connect LLMs to multiple data sources and knowledge bases empowers enterprises to interact with data in a more conversational, intuitive manner. NeMo Retriever is poised to enhances user experience and drive efficiency and productivity across various business functions across enterprises.
Developers eager to leverage this revolutionary technology can sign up for early access to NVIDIA NeMo Retriever.
The excitement surrounding this release is palpable, as it enhances current AI capabilities and opens up a host of possibilities for LLM adoption in the enterprise sector. The term “game-changer” gets thrown around more often than not in recent AI news. Still, NeMo Retriever is a keystone in linking generative AI and actionable business intelligence, providing a tangible bridge between advanced AI capabilities and real-world enterprise applications.
Engage with StorageReview
Newsletter | YouTube | Podcast iTunes/Spotify | Instagram | Twitter | TikTok | RSS Feed